Role of NLP in Customer Service
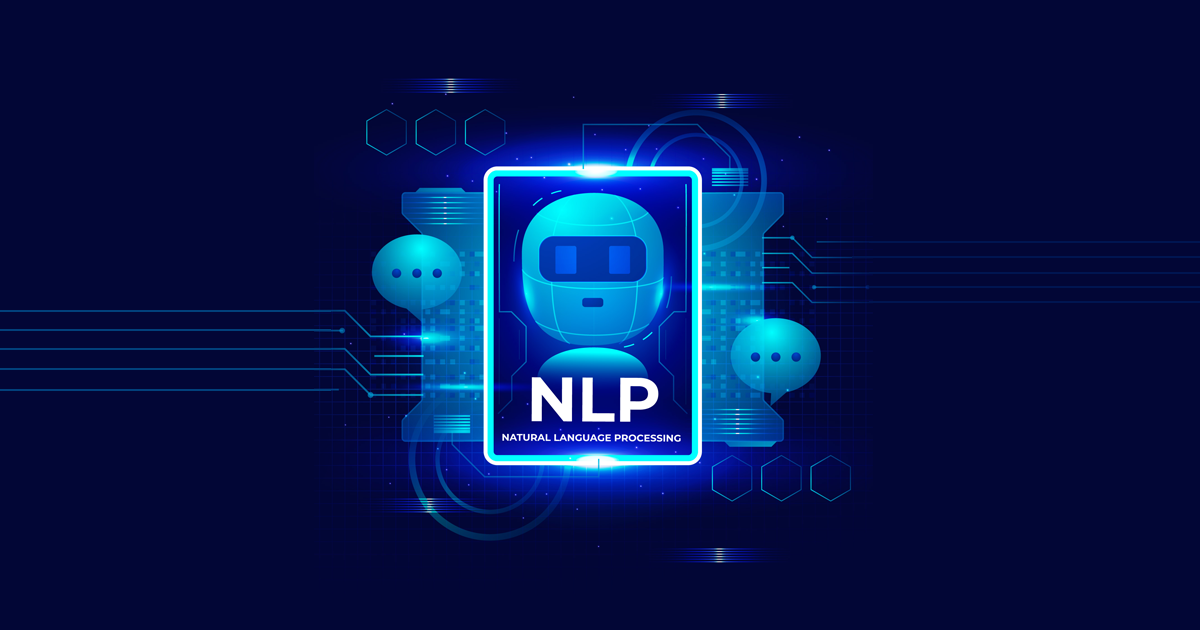
What is The Role of NLP in Customer Service?
Natural language processing (NLP) has been effectively applied to a wide range of applications, including data processing, virtual assistants, manual processing and control systems. Despite its many applications, NLP may be best suited to sales and support. Human users may communicate with software in real-time while browsing and purchasing online, thanks to smart chatbots that can be found on websites and order forms all over the world.
In the field of customer service, where the innovative use of technologies is utilised to support customers, guide operations, and enhance sales, natural language processing (NLP) has become an indispensable tool. Let's look at how NLP is crucial in the field of customer service.
How is NLP Helping The Customer Service?
1. Chatbots for Customer Service
Chatbots are used in a variety of applications, including customer service. In recent years, they've made quite a sensation. In terms of chat platforms, Facebook and WhatsApp each have over 1 billion members. So, as Hendrik Luuk, CTO at Alphablues, notes, "it's evident everybody will be utilising some sort of chat platform eventually." And as more consumers spend their time chatting, this has an impact on businesses. The bulk of customers nowadays communicates with a company via chat. Instead of hiring more employees to handle these inbound consumer inquiries, NLP and NLU (natural language understanding), a subset of NLP, can be used to automate the discussion. In this sense, using chatbots and natural language processing to automate your customer service can significantly reduce your expenditures. Another advantage of using NLP to automate chatbots is the capacity to scale your customer care.
2. Advertising and Marketing
If consumer demographics and usage patterns were once the primary emphases for marketers, they are now swiftly adapting to the new digital world. NLP helps businesses generate individualised and specifically targeted marketing strategies by analysing digital footprints such as social sites, emails, search phrases, and browser behaviour. NLP technology allows social prospecting to filter through mentions on social media and determine to buy intent. Typically, NLP employs keyword matching to extract expressions of interest and profiles of persons who meet the customer's requirements. However, the most recent NLP technology also considers the situation in which the keyword was uttered. Customers are shown material that they are truly interested in in this manner.
3. Reputation and Sentiment Analysis
The internet and digital media are valuable resources for businesses to learn about their customers' needs, opinions, and intentions. NLP allows businesses to listen to and comprehend their consumers' online voices, as well as the sentiment behind them. Customers' choices and decisions are influenced by the mood and emotion shown in online conversations. Companies may now search the entire internet for references to their brand and products and take appropriate action. Businesses benefit from this because they can better comprehend their clients and make more informed business decisions.
What are The Natural Language Processing Techniques Used in Customer Service?
Check out the 5 most common Natural Language Processing techniques which are used in customer service:
1. Named Entity Recognition
Retrieving the elements in the text is the most basic and important technique in NLP. It illustrates the text's most important ideas and references. Named entity recognition (NER) extracts entities from text such as individuals, places, organisations, dates, and so on.
2. Sentiment Analysis
Sentiment analysis is the most extensively used NLP approach. Sentiment analysis is especially beneficial in situations where people share their opinions and feedback, such as survey responses, ratings, and comments on social media. In more complicated circumstances, the result can be a numerical score that can be categorised into as many categories as needed.
3. Text Summarization
As the name implies, NLP approaches can be used to summarise vast amounts of text. The most prevalent uses of text summaries are in news articles and scholarly papers.
Abstraction and Extraction are two methods of text summarization. Extraction methods extract elements of the text to construct a summary. Abstraction methods generate new text that expresses the essence of the original material, resulting in a summary.
4. Aspect Mining
Aspect mining is a method for detecting various features of a text. It pulls comprehensive text information when used in combination with sentiment analysis. Part-of-speech labelling is one of the simplest methods of aspect mining.
5. Topic Modelling
One of the more difficult ways for identifying natural subjects in a document is topic modelling. Topic modelling has the advantage of being an unsupervised technique. There is no need for model training or a labelled training dataset.
What are The Challenges in Natural Language Processing?
However, NLP is still in its infancy, and data scientists face numerous obstacles. According to Katie, one of the most difficult aspects of NLP is accurately expressing language data. Given that human language is so thick, with numerous levels of meaning ranging from the tone with which a term is said, to the cultural setting, to the varied styles of writing used for different purposes, it is extremely difficult for NLP to absorb all nuances and essential information. So, if you're lousy at detecting sarcasm and trying to develop a model to classify it, you're not going to make much progress since machines are terrible at making social evaluations.
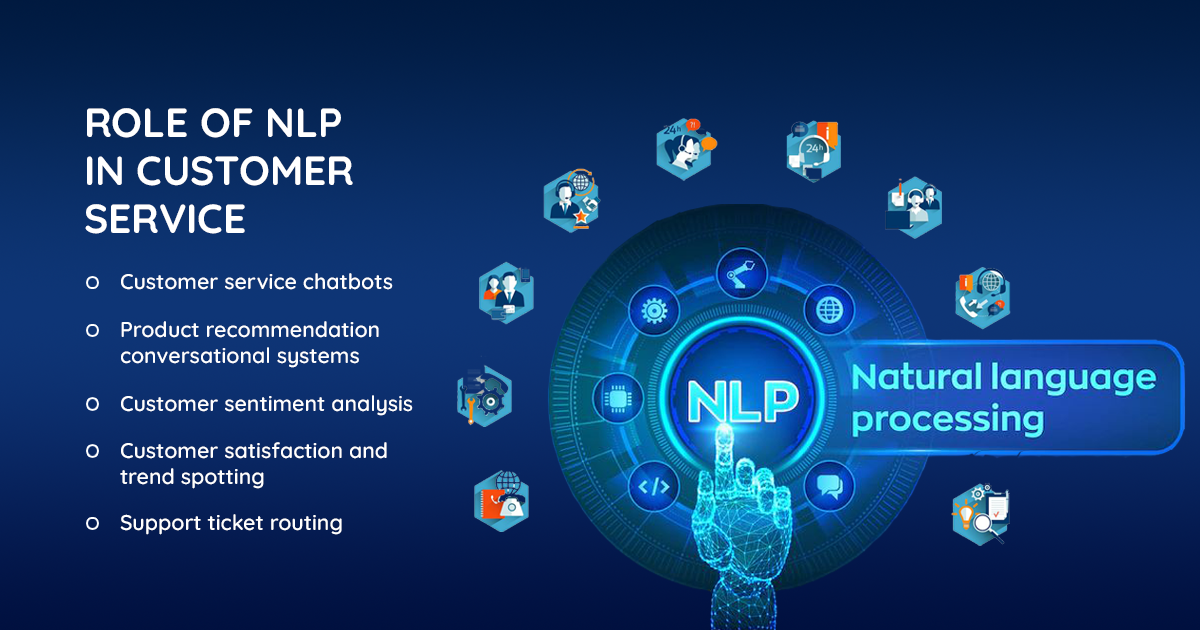
Aside from recognising social context and sentiment in language, NLP also faces the following challenges:
- Breaking the sentence, also called "sentence boundary disambiguation," is the act of dividing a paragraph into proper sentence units by a machine. It's a crucial procedure because it's difficult to separate recognised sentence sections when we have very complicated data in the form of tables, illustrations, notations, and page breaks. This type of information should be examined so that the machine can make sense of it.
- Part of speech (POS) tagging – There is no need to distinguish distinct pieces of speech or text when individuals communicate since they innately comprehend the meaning of the communication. When a computer learns, though, it must formally comprehend where each word in a sentence, phrase, or document belongs.
- Setting the context is among the most difficult and crucial tasks in the NLP process since it includes training a machine on how to extract context from a portion of the text. When coping with homonyms, such as "a bank" as a financial organisation and "a riverbank" as a riverbank, the computer should be taught to recognise that the environment of the two is distinct.
- Understanding the meaning of semantics – For a system to apply gained knowledge, relying solely on linguistic analysis is insufficient. A system must grasp the semantics of vocabulary phrases in the text in order to correctly apply knowledge. If two words come in the same setting, but the total information of the sentence differs due to semantic differences, this is an example.
Conclusion
NLP has a big future in improving the customer service sector of many companies. As natural language processing (NLP) advances, it is altering the way customers interact with brands. However, it broadens the range of information that businesses can obtain about their clients. Furthermore, businesses may hear what customers have to say in their own words. They can then build more appropriate plans based on the data.