Machine Learning In Cybersecurity & Blockchain
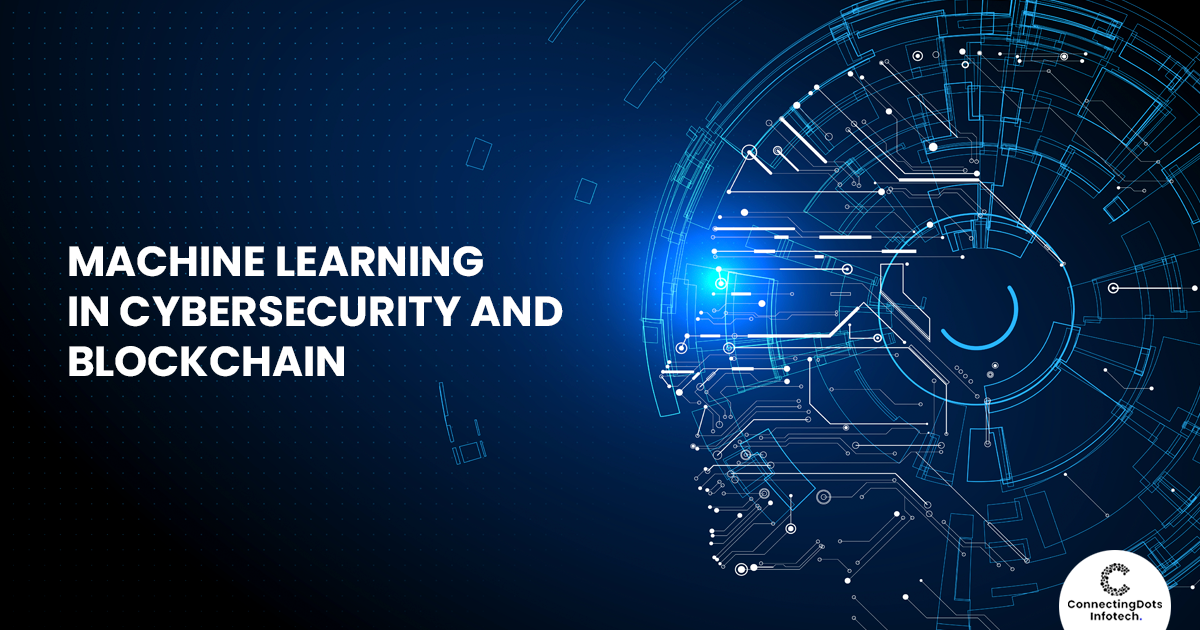
People use Machine learning to interact with the world around them nowadays while using the internet, mapping, e-commerce, emailing, social networking, and even while playing video games. Smart home security systems, medical technology, remote-controlled (driverless) automobiles, and picture and speech recognition, among other fascinating future applications of machine learning. Blockchain technology has so far been widely used in the cryptocurrency industry.
Future developments in the blockchain industry are expected to include decentralisation and distribution of power and financial industries with top-notch security. It gives users simple access to diverse assets, information, and cash in the form of technology and gives users control and authority over those things. From a security standpoint, ML and blockchain-based clouds are working together to provide the best big data protection.
A list of recent technologies that aim to provide cyber-security is now provided in the edition of cyber-security. These solutions are made possible by gamification, blockchain-based cloud computing, artificial intelligence (AI), machine learning (ML), and other developments. These cutting-edge solutions provide enterprises with defense against cyber threats, data breaches, and phishing, as well as against sophisticated attacks hidden in the cloud, endpoints, and network layers.
Machine learning is Used to Prevent Attacks and Detect Malicious Events
Machine learning algorithms will allow firms to recognise malware and stop attacks before they start. David Palmer, the director at the UK-based technology company Darktrace, claims that since its founding in 2013, the business has been effective in implementing ML-based immune solutions.
Recent data exfiltration attacks performed via a linked fish tank that took place in a casino store in North America were discovered by Darktrace using the ML algorithm. The business claims to have thwarted an attempt akin to the well-known WannaCry ransomware disaster, which affected more than 200,000 people across more than 100 nations. Palmer claims that their ML-based algorithms quickly identified and stopped the intrusion in the NHS agency network.
Mobile Endpoint Analysis with Machine Learning
Although ML is being more widely used to develop mobile devices, the focus of this endeavour has been on voice-activated programs like Google Now, Siri from Apple Inc., and Alexa from Amazon.
Security firms are attempting to help organisations embrace machine language-based mobile antiviral technologies. For instance, MobileIron and Zimperium have partnered to sell their combined alternative that can identify the device, connection, and application attacks and automatically ameliorate the action to offer data security. Zimperium's ML-powered cybersecurity is to be incorporated with MobileIron's security and compliance turbocharger.
Utilising ML to Strengthen Human Analysis
Machine learning is thought to help human analysts with a variety of tasks, including malware detection, network analysis, endpoint security, and vulnerability analysis. For instance, the Computer Science and Artificial Intelligence Lab (CSAIL) at MIT developed an AI system. This machine-learning platform helps security analysts find the "needles in a haystack" in 2016. This technology could, among other things, filter the data, send the data to a human analyst, and review millions of logins every day.
Machine learning is Employed to Automate Repeated Security Tasks
The firm's workforce will concentrate on other crucial problems by automating monotonous tasks. The ultimate goal of ML is to eliminate the need for humans to handle routine, low-value decision-making tasks.
For instance, the information technology consulting company Booz Allen Hamilton has apparently embraced this strategy, employing ML technologies to effectively replace human security resources so that personnel can concentrate on other activities.
Zero-Day Vulnerabilities are Fixed by Machine learning
Particularly with regard to zero-day threats that target unprotected IoT systems, ML can help close vulnerabilities. On this, security experts are actively working. For instance, a team of professionals at Arizona State University has created anThe.
The ML-powered solution to examine traffic in DARK WEB and find information connected to zero-day exploits.
The advent of cryptocurrencies is a direct result of the pressing need for contemporary financial solutions. Recent blockchain research demonstrates that electronic money can be safely transferred between peers without the involvement of a third party.
It functions as a ledger for transactions and provides security against hackers when using virtual currency. According to the rules, the transaction records are encrypted and executed on computers using blockchain tools. The fact that different parties own the transaction data makes it difficult to attack and reduces security costs. Through widespread participation, the transactions are approved, and promptness is guaranteed. Additionally, using an open source makes it simple to implement, connect, and expand the system. Records of the transaction can also be publicly disclosed and accessed, allowing for the public disclosure of all relevant information while saving money on regulatory costs.
Using blockchain provides more security than keeping the data in a single centralised database. Data harm caused by attacks is avoided in terms of data management and storage. Additionally, the data can be made available to any authorised party upon request because of the blockchain's transparency. These advantages make it suitable for a variety of industries, including the financial sector and IoT contexts.
Combining these transactions over a network to form a block allows someone to finalise transaction records through an authentication process. Due to their widespread accessibility and effectiveness, cloud computing and blockchain are employed together. The changes indicate that additional decentralised cloud service providers will emerge in the future and will undoubtedly compete with the current ones as blockchain-based decentralised cloud security solutions are still in their infancy.
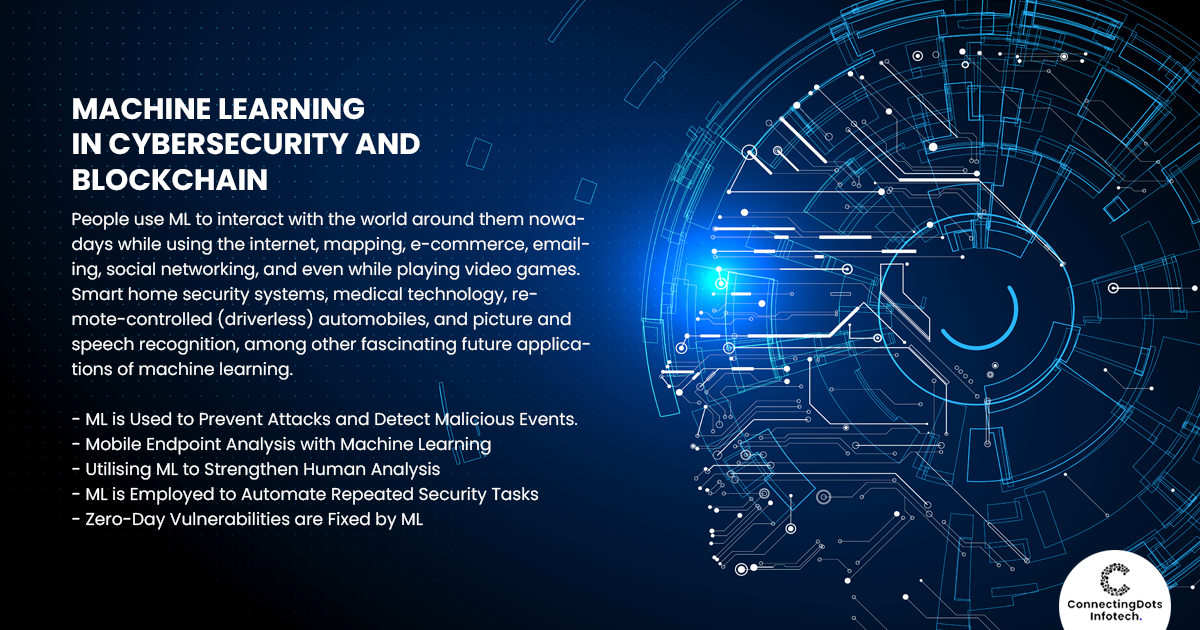
Conclusion
ML and blockchain-based cloud security solutions can provide your data with top-notch security capabilities, but they are not a panacea. For instance, the algorithms might not be helpful if they are poorly developed. This indicates that the technologies must be appropriately constructed in order for them to operate effectively.